.png)
Data Talks on the Rocks 8 - ClickHouse, StarTree, MotherDuck, and Tobiko Data
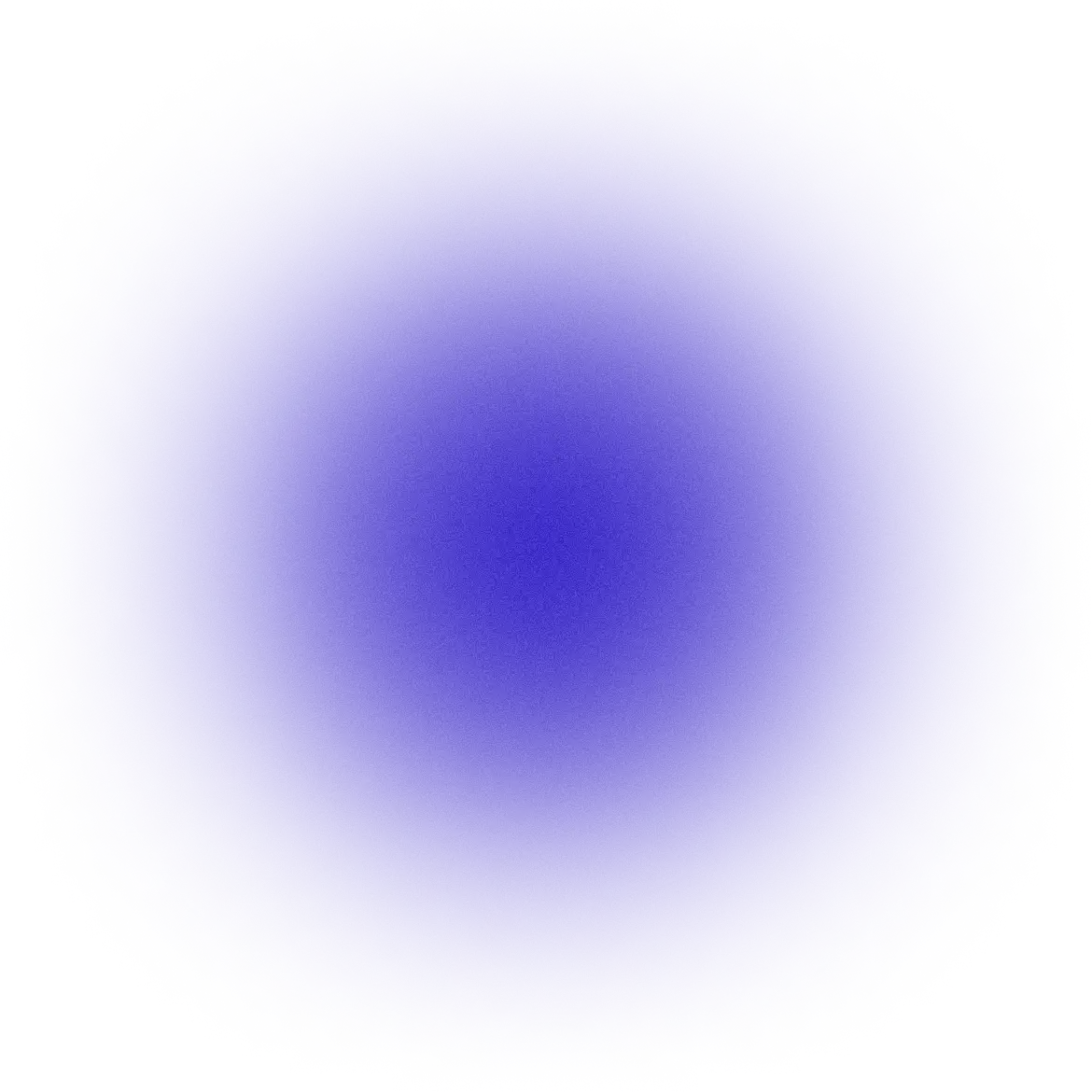
Data Talks on the Rocks is a series of interviews from thought leaders and founders discussing the latest trends in data and analytics.
Data Talks on the Rocks 1 features:
- Edo Liberty, founder & CEO of Pinecone
- Erik Bernhardsson, founder & CEO of Modal Labs
- Katrin Ribant, founder & CEO of Ask-Y, and the former founder of Dataroma
Data Talks on the Rocks 2 features:
- Guillermo Rauch, founder & CEO of Vercel
- Ryan Blue, founder & CEO of Tabular which was recently acquired by Databricks
Data Talks on the Rocks 3 features:
- Lloyd Tabb, creator of Malloy, and the former founder of Looker
Data Talks on the Rocks 4 features:
- Alexey Milovidov, co-founder & CTO of ClickHouse
Data Talks on the Rocks 5 features:
- Hannes Mühleisen, creator of DuckDB
Data Talks on the Rocks 6 features:
- Simon Späti, technical author & data engineer
Data Talks on the Rocks 7 features:
- Kishore Gopalakrishna, co-founder & CEO of StarTree
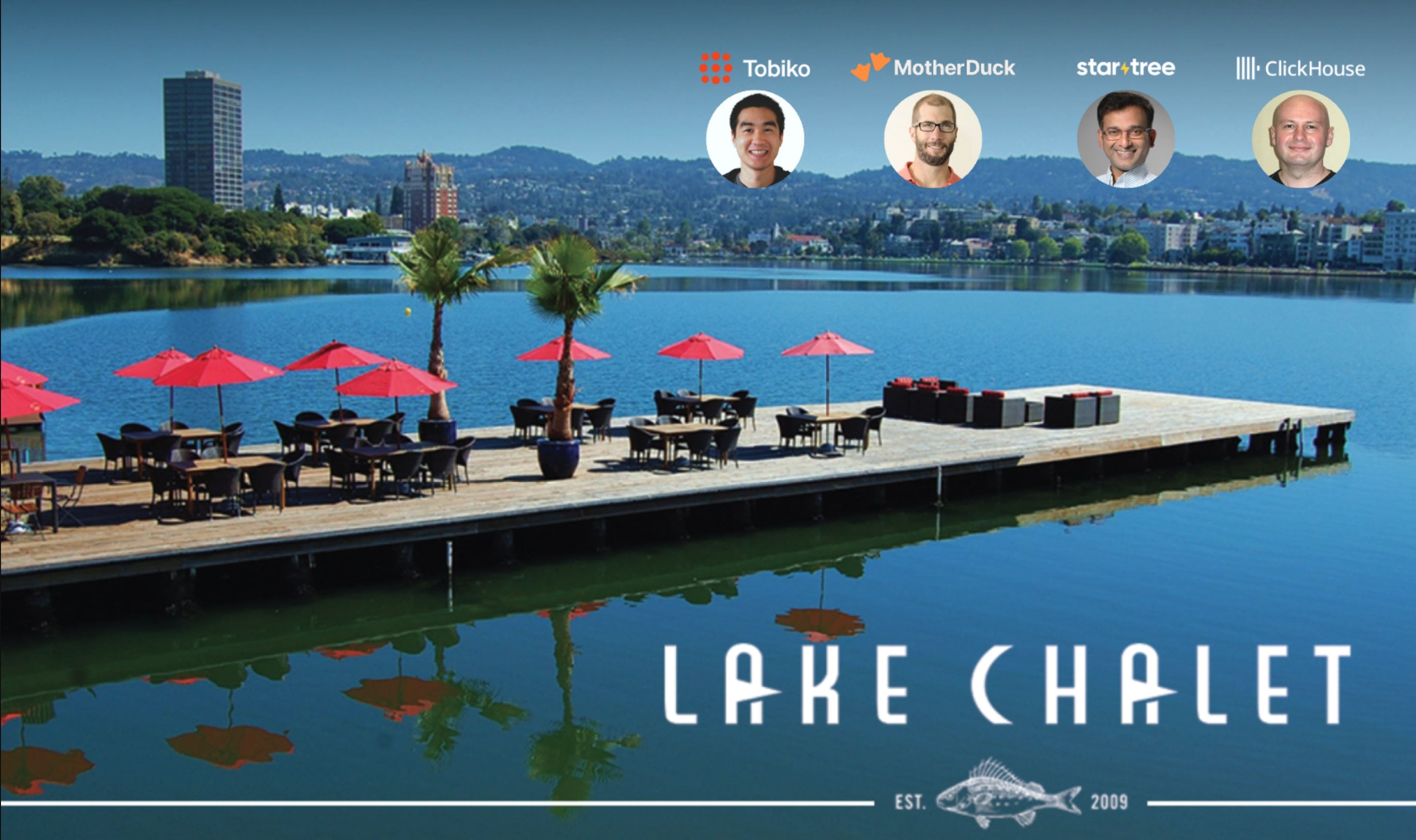
On April 23, 2025, 200+ data practioners and technical leaders joined our all-star event during Data Council. We discussed real-time databases and next-generation ETL with a legendary panel of technical founders Yury Izrailevsky (co-founder of ClickHouse, ex-Google, Netflix, Yahoo), Kishore Gopalakrishna (founder of StarTree, created Apache Pinot at LinkedIn), Jordan Tigani (co-founder of MotherDuck, ex-SingleStore and Google), and Tobias (Toby) Mao (founder of Tobiko, creators of SQLMesh and SQLGlot).
I’ve noted some of my favorite highlights below:
(quote 1)
(quote 2)
(quote 3)
(quote 4)
Real-time database roundup with the founders of StarTree, MotherDuck, and ClickHouse
Michael Driscoll
I am a huge fan of real time analytic databases, warehouses, query engines. For background, I worked at a company before starting Rill that actually created one of these databases called Apache Druid. It's fair to say I've watched from the sidelines while all three of these gentlemen have been building businesses with some amazing technology that I can honestly say are superior versions of the real time analytics database that we created ourselves. So, hopefully we inspired some of those architecture choices or inspired some false lessons about what not to do with our own work.
So to introduce the folks I've got here, I'm going to start on my right. I'm delighted to have Yury, the president and co-founder of ClickHouse with me. Yuri has done time. I can say he's done time at some of the largest and most successful companies, here in Silicon Valley, most recently, Google, but also before that, Yahoo, where he overlapped with Kishore. Next we've got Kishore, who is the founder and CEO of StarTree, the commercializers of Apache Pinot. Kishore worked at LinkedIn, where he actually invented Apache Pinot and then, went on to start StarTree which we'll talk more about a bit. And finally, certainly last but not least, the only non-Yahoo of the three who's actually, believe it or not, Jordan, we found out we were classmates many years ago. We graduated the same year. We probably met at some point. But Jordan is the co-founder and CEO of MotherDuck, the commercializers of a DuckDB cloud offering. And before that, Jordan was running product at a company called SingleStore. Before that, very involved at Google. Again, overlapping with Yury has been everywhere, working on BigQuery.
So we're going to get into it. The first question I've got for this distinguished panel. Databases, the joke is every database company is an overnight success after seven years. I'm going to start with Jordan, maybe you're the most recent, we're going to go back. Jordan, if you could tell us what was going through your mind when you thought it was a good idea to found yet another database company? And I'll ask to add a layer to that: what were the gaps in the market that you observed? And what gave you the courage and confidence to go out and ask folks like Martin [Casado], and some of your initial investors to write big checks to create and found yet another database company.
Why start another database company? What were the gaps in the market?
Jordan Tigani (03:00)
So one of the things that I'd been noticing was, I'd worked at BigQuery for ten years, and we had some of the largest customers in the world. We had Equifax, Home Depot, Walmart, and I dug into how people were actually using their data. And the vast majority of customers using BigQuery didn't have big data. The ones that did have big data, they only used small slices of data at a time. And, so I kind of filed that away. I worked at SingleStore for a couple of years, and I just sort of noticed that people were asking us for smaller instance sizes. And then we spent all this time building this distributed infrastructure, and some of our most successful customers were actually ones that were just running on a giant box. I think Sony was one of the largest customers, and they were running like these massive boxes, and they never had any problems. And it was like, wow, that's, you know, there's something to it. They never have any problems. It just works. And kind of what the shape of modern hardware is different than when we started building BigQuery. And then I saw this database, you know, this open source database started showing up in some benchmarking reports. They were giving us a run for the money. MemSQL, SingleStore, our whole thing was speed, we're going to do whatever it takes to be fast. And then this sort of random couple of graduate students, you know, academics in the Netherlands were like we're getting pretty close out of nowhere. And I'm like, huh, somebody should really take that and build a serverless, cloud version of it. And I'm like, hey, I've built two SaaS databases. Maybe that should be me and kind of thought about it a little more. And, I sort of tripped and fell and, Martin [Casado], offered me a term sheet and I realize that not every founder's fundraising journey is that way. But it's sort of that I stumbled into starting a database company.
Michael Driscoll (05:05)
Okay, I have a follow up question, but I'm going to go down and ask the same question. So Kishore, same question. What was the gap in the market? When you started StarTree, Apache Druid was out there, ClickHouse I think did exist and was open source at that point. What motivated you to have the courage and the confidence to go and actually start StarTree and try to build yet another database business?
Kishore Gopalakrishna (05:33)
Before getting to that, I do want to answer your previous question on why did we build in the first place. I think, to be honest, I actually didn't want to build another database. I had built one before this, which was at MongoDB called Espresso. It's very hard, as you said. And I think you mentioned it takes seven years. I think it takes seven years to realize that it's hard. So I think that's when you really get into the details of what's the database. I think for us it was kind of completely opposite of what Jordan was actually talking about.
For us at LinkedIn, we were actually going in the other direction of scale right now. I think most of you know “who viewed my profile” on LinkedIn, right? That's kind of one of the things that actually drove us to build this, which was there are 5000 people looking at it on any given second, and that was not really something that people thought about analytics and this kind of a workload on top of that. So for us, we were seeing this kind of OLAP functionality being needed at OLTP scale. So that's kind of what got us excited as well, that, hey, these are two hard things. On one side, you have tons and tons of transactions coming in at the same time, you want to provide rich analytical functions. And the second part was also who you were serving. I think the Who was actually a very important part for us. It was not internal members of LinkedIn, or within the company. This is really the main users of LinkedIn. So when you actually start providing…
Michael Driscoll (07:05)
User facing analytics.
Kishore Gopalakrishna (07:07)
User facing analytics. So that's kind of the term that we coined, which is really very different when you actually get into the weeds of it. Your users don't want to wait for a load page to load for seconds, right? I mean, it's okay in the data warehouse, internal use cases because you don't have the option. But when you sell something to your end users, you have to be in milliseconds. And for us, we were actually having P99 latencies and alerts on that. So very different requirements. And that's kind of when we said most of the existing databases were not catering to that workload characteristics. And we said like this is it. We need to approach it from first principles, go back to see like, hey, what can we do to reduce the work done per query. I think that was our key thing versus just doing it faster.
Michael Driscoll (07:52)
Yury. The same question. You could have just been a comfortable guy. You were a VP at Google. You know, it's a great place to just collect it. Not to say it's an easy place, but you decided or someone convinced you to say, you know, Yury, do you want to just be an important big guy at Google, or do you want to get into the weeds of a brand new startup and start from zero and create a database business? What convinced you that ClickHouse should be another database company?
Yury Izrailevsky (08:30)
So I was a Google when I first heard about ClickHouse. I was actually working together with the best dressed man in the room, with my colleague Jordan. We were having a great time in Google Cloud. But yeah, the first time I heard about ClickHouse, by the way, disclaimer: I did not create ClickHouse originally. It was first created, back in 2009 by Alexey Milovidov of one of my co-founders and open sourced in 2016. And then I first heard about it around 2018.
Michael Driscoll (09:06)
He created it in 2009?
Yury Izrailevsky (09:08)
2009 in-house. It was incubated at Yandex. I first heard about it actually from my little brother who was working at Uber at that time, and they were having issues scaling their analytics platform. They were using Elastic and Splunk and some other things, and they did a bake off and they tried all sorts of different databases and technologies to see which one would work best. ClickHouse was by far and away the best system. They just outperformed everything else head and shoulders above. And I remember him calling me.
He was like, Yuri, what do you know about ClickHouse?
I don't know anything about ClickHouse, what is it?
He said check it out, it's really, really good.
And I remember I downloaded it on my laptop, I installed it and started running queries, and I think I thought things were broken because it was so fast. It just comes back right away. It's like, this can't be right.
So, I filled up my entire laptop hard drive with data and I kept running queries and it still kept coming back immediately in real time. Wow, this is really amazing. And then over the years, I kept hearing more and more. And companies like Uber and eBay and Microsoft and others just kept adopting it and talking about it. And then in [20]21, when an opportunity came up to take the IP and bring over the team of original ClickHouse developers, and build a business around it and create a cloud service around it. You know, I just.
Michael Driscoll (10;45)
It was a no brainer.
Yury Izrailevsky (10:47)
It was a no brainer, right? Like when you start with really, really great technology, everything else is okay.
Michael Driscoll (10:52)
So it was more than seven years that ClickHouse had been baking.
Yury Izrailevsky (10:57)
I would say more like ten plus years to really harden database technology. And again, you can't take risks. This is data. The most valuable thing that any of us have. Well, other than our children of course.
Michael Driscoll (11:11)
So, if our children are listening, you're the most valuable thing out there. So they know.
Yury Izrailevsky (11:15)
And so, yeah, you really, really want something that's battle hardened and can be trusted.
Small Data Movement
Michael Driscoll (11:21)
So you talk about the opportunity to create a cloud service. And then in terms of lineage and origin story, it's fair. Jordan, also, I think I'd love to ask you this question, which is, tell us a little bit. Similarly, you are not the creator of DuckDB. Certainly, the creator of the leading commercial business that is using DuckDB. I think the key question that a lot of folks have is, there's an open source product and then there's this cloud offering and I'd love to hear from you. And I'm going to maybe add a layer. Another question is that I've heard about the small data movement and the thing that I think about when I hear small data, is if someone pitched me on a banking idea. We looked at all of our customers at banks, and we found that, you know, most customers don't have a lot of money. So I'm going to build a bank for small balances. I'm going to build a bank for people who don't have a lot of money. I don't know that I would, as an investor, get excited about a bank for small balances. And when they get big enough, they'll go to JP Morgan. And, you know, I’ll lose their business. But that's a side question, what is the difference between the architecture that people love running locally on small data, and certainly that inspired you to build? And what MotherDuck is doing in the cloud?
Jordan Tigani (12:41)
So first of all, I think it'll be an awesome idea to build a bank for small balances, because the vast majority of people have small balances, and the vast majority of banks, all they really care about is their customers that have a shit ton of money. And so I think you could have a great user focused, banking experience that could do really well. And I think actually that ties into what we want to do at MotherDuck. Yes, there are use cases for lots of data. And yes, some people have lots of data, but I've built software systems for 20 some years. And as you're designing a system, if you have a 99% workload and a 1% workload, if you design your system for the 1% workload, then you're going to totally skew how that thing works. So you want to design for the common workload and make sure the other thing works. And so with DuckDB being designed for a single node scale up, it works super well in that mode. And with modern database techniques where you page data out when it's not being used, you can handle the giant workloads. But then you can also give an amazing, amazing, experience on the smaller use cases.
So you also asked about how you deal with the open source versus the SaaS version. So originally I went to Hannes and Mark, who are the creators of DuckDB and I said, like, hey, you know what? You guys have built something amazing, will you give me a job? I'd love to sort of work with you and build a SaaS service. You're probably building a SaaS service. And they said, we don't want to build a SaaS service. We just want to focus on building this amazing database. But we'll partner with you. That sounds interesting. And so, I ended up talking to some VCs actually later in the same day, who then turned around and wanted to invest. But I think the other key thing was like, we didn't want to just say hey, you guys built an amazing database, we're going to go, kind of take that IP and try to make a bunch of money out of it. So we gave them a co-founder share in the company as we did it they also gave us an exclusive. They weren't going to work with anybody else that was competing with us. Then we also funded some of the development of DuckDB, which I think is actually a really, really nice and a stable, division of labor between open source and closed source, because, you know, we are unapologetically, a company that wants to make money, and our investors would like to hear that because they've invested a decent amount of money in us. But DuckDB Labs, they get to focus on the ideas of building an amazing database. They can be pure, they get to not worry. Like there's no pressure on them. We can put pressure on them to change the license. You know, the license, it's owned by the DuckDB Foundation. If we told them we wanted it in order to change the license, they would tell us, you know, something not very nice.
Michael Driscoll (16:06)
Get lost. Something in Dutch that you don’t understand.
Jordan Tigani (16:10)
Or some area in German that would sound even filthier. But I think it's great. And if things work out for us, then it also works out for them and then they get to just focus on the thing that they love doing. And I'm hoping that this becomes, if it works out for us, it'll be a model that other people can follow in the open source world. If it doesn't work out, it'll be a cautionary tale.
Michael Driscoll (16:41)
But they'll be learning either way.
Jordan Tigani (16:42)
Yeah, exactly.
Pricing Models
Michael Driscoll (16:43)
So, Kishore, one of the things that people often ask when it comes to the way that open source companies monetize when they build a commercial cloud offering is the licensing model or the consumption model. What I've heard when I've talked to some of your customers who are using StarTree to leverage the power of Apache Pinot is that I had heard that you had a pretty unique licensing model, that was different from what was being offered from others. Tell us a little bit about what that model is. I guess, to frame it. I often think of two models, actually two axes. One is a consumption model, which is just like a Snowflake model. They have those magical Snowflake credits that you have no idea what they mean. But you're consuming them and they cost a lot of money. Then you've got a licensing model, which is, obviously you pay, all you can eat. Maybe that’s seat based, so that it's company wide. What model has StarTree found to be most appealing to your customer base and why?
Kishore Gopalakrishna (17:50)
Yeah, I mean, obviously, from a company's point of view, everyone loves the Snowflake model because you take the credit.
Michael Driscoll (17:58)
The investors love it.
Kishore Gopalakrishna (17:59)
I mean, the company, everyone loves it, but the customers don't really like it, right?
Michael Driscoll (18:04)
You're right. Everybody but the customers. But hey, who here, who cares about the customers?
Kishore Gopalakrishna (18:08)
So I think what happened when we started, we actually tried to talk to the customers and see what is the thing that they actually love, right? And I think at that point, most of them were burned by the Snowflake model because they take it and then everything gets used up very quickly. And I think there was also another part of it, which was the incentives are actually not lined up. And if you kind of put yourself in Snowflake's position. I don't want to make my database faster, because if I make my database faster, my customers will be using less credit.
Michael Driscoll (18:43)
So drop or place sounds great. Maybe dbt is a great ETL framework. We just keep dropping and replacing these tables.
Kishore Gopalakrishna (18:50)
So in a way inefficiency is slightly better there. So that's why our model was actually slightly different because we wanted our customers to run as many queries as possible, because that's really where the true value is. And we wanted to say you should be running thousands and then hundreds of thousands of queries per second. And that's where we went into the other model, here is what we can actually prove. A single core can actually do it. So that's why we went to the core model and say like, yeah, as long as you have this core, you can actually run as many queries as possible and then continue to ingest as many events as needed as well. So we didn't want them to be thinking about, oh, I have to pay per query per event. It is just a very complicated model for it to be reasonable. And we wanted to prove that, hey, with a small number, you can actually do a ton of queries. And for us, that was really the value that we would see from our customer side is how many queries are you running on a per second basis. And then we have the data in Snowflake took probably like ten years to get like 1 billion queries per day. We were there in three years, across our customers and a 10x model compared to the…
Michael Driscoll (20:02)
1 billion queries a day, meaning 1 billion queries across their customer base. Interesting.
Kishore Gopalakrishna (20:06)
So, that's kind of what we really want to show all that you can. I mean, if you just look at LinkedIn today it is running at 600 million queries per day, just LinkedIn.
Michael Driscoll (20:17)
So that means Snowflake's making a lot of money per query.
Kishore Gopalakrishna (20:19)
Exactly. I think that's the key idea. And if you look at it, our goal is to really reduce that cost per query model. And this is again going back to what Kafka did at LinkedIn as well. And that's kind of why people are logging a lot of events today. It's because the cost per logging and event is actually drastically reduced compared to the prior models. So that's what we wanted the people to be running a lot of queries, using the data.
Michael Driscoll (20:46)
Without worrying about getting charged right per query. Okay. So Yury, I know that the ClickHouse, I guess it was maybe 18 months ago, maybe a year ago. You had a very expansive, discussion, exposition of the architecture of ClickHouse Cloud. I would say as an observer, one of the disadvantages sometimes of having a great open source product as a core technology is that it's so great that people are like, well, gosh, we just like ClickHouse is amazing. They had that same experience you had. It's so fast on my laptop. Maybe give us a sense of, I guess two questions. One is what is the architecture of ClickHouse Cloud? And in particular, what did you choose in the architecture to drive differentiation? What differentiates ClickHouse Cloud from running ClickHouse on a really beefy, huge machine on Amazon or Google and what's the architecture of the cloud offering that allows for that differentiation?
Architecture Deep-Dive
Yury Izrailevsky (21:52)
Sure. It's a great question. So I guess if you take a step back and you just look at the idea of open source being free software, why on earth would you pay a company for something you can get for free? And there are many, many reasons why you would want to do that. So when people deploy ClickHouse open source, self-managed, deployment, typically, it's using a shared-nothing architecture where the data sits directly on the nodes. It's much simpler this way and it can be pretty fast. But the downside is it's very rigid because there's a lot of data and metadata to maintain and manage. It's very hard to do upgrades. It's very difficult to scale the cluster up and down, replace the nodes if something fails. So it's a very, very rigid structure. You have to overprovision for your peak loads and you have to pay for it 24/7.
In the cloud, when we first created the company and decided to build a cloud product, we chose a very different architecture under the hood. Again, the query is functional, it's the same thing, the same queries. You run an open source, you can run in our cloud product, but under the hood it's a completely different architecture. So we moved away from shared-nothing to compute and storage separation where the data sits in object storage like S3 or GCS, and the compute nodes are completely stateless. We're not keeping any data or even metadata on those nodes. Then of course we do caching and pre-fetching, all of that stuff to make it fast. But it's not required that the data sits there. And as a result, it allows you to do scaling up and down instantaneously. You can do idling, you can even do things like compute compute separation where you have different compute clusters for different use cases, like separating reads and writes, or different organizations who don't want to step on each other's toes or have different budgets. You can do all of that with this architecture. And because it's serverless, it scales up and down. You only pay for what you use, and that actually ends up being cheaper. So not only is it more reliable because it can scale up to whatever your peaks are, it scales with the elasticity of the underlying cloud provider, but it is also cheaper for you. And on top of it, our cloud product, we've added a whole bunch of security features like, identity, cloud native identity, and access management, RBAC, bring your own key. We can do FIPS 140-2 builds. There's a whole slew of certifications. ISO SOC2 Type 2, PCI, HIPPA, working at FedRAMP and so forth. And of course, it's a fully managed product, right? It's got a great console. We do backups, restores. There's a nice SQL editor with an AI agent, a semantic interface, you just tell it what to do. And it does a great job building query. So it's a much, much nicer experience. It's like being in a self-driving car. It just takes you wherever you need to go. You don't need to worry about it.
Michael Driscoll (25:13)
The enthusiasm is fantastic. It's like Elon telling us about the full self-driving cars that are going to be running our entire taxi fleets.
Yury Izrailevsky (25:26)
But I'm 100% objective. No bias at all.
Michael Driscoll (25:29)
But I will say on the security features, we do know that DeepSeek, probably could have used some of those security features because they had a leak on their ClickHouse cluster, what was the result of that leak? They just gave away all the prompts or something.
Yury Izrailevsky (25:45)
It was nothing. As far as I can tell.
Michael Driscoll (25:48)
It's complimentary that they were using ClickHouse, but they were not using ClickHouse with security.
Yury Izrailevsky (25:52)
It's interesting. Well, they were not using ClickHouse Cloud at all. They were using open source and they actually went out of their way to open up some of those ports that by default are not open to the internet. So they shot themselves in the foot. But yeah, had they used ClickHouse Cloud they would have been much better off.
Thoughts on the Lakehouse
Michael Driscoll (26:10)
Well, we'll see if you get an inbound marketing request for a demo from those guys in the coming days. One of the things you talked about with the architecture of ClickHouse Cloud is that separation of compute and storage. Certainly, that was the innovation that Snowflake touted for why they were able to kind of take the traditional warehouse market in the way that they did. The topic that's on a lot of our minds or the technology that we hear so much about these days is Lakehouse. Here we are at the Chalet. I wish it were a little nicer out. But we are in an actual lakehouse, so I would be remiss if we didn't talk about lakehouses. Amazing marketing term. Whoever came up with it. So someone at Databricks deserves a promotion for that.
Yury Izrailevsky (26:57)
ClickHouse had nothing to do with it. Although it fits perfect. ClickHouse Lakehouse.
Michael Driscoll (27:04)
Well you know we have one house and another database company out there. But Jordan I've heard from folks in the DuckDb world that this is the year of the Lakehouse. Tell us a little bit about Iceberg. This is the technology that Databricks paid $2 billion for Tabular, the guys behind the Apache Iceberg project. How is MotherDuck embracing, complementing, working with this, what many of us feel is the future substrate of all data?
Jordan Tigani (27:38)
So, first of all, I'm perhaps a little bit of a lakehouse skeptic. I think that there are, you know, I built the storage system for BigQuery. And so I kind of have been deeply involved in the data warehouse storage for a long time, and there's a lot of benefit to being able to decouple the query engine and the storage layer. And you probably lose a factor of at least three in order to do that. Maybe that's fast enough. Maybe that's fine. I also think that there's a bunch of things that you give up in the security realm, in the metadata management, and fast updates and transaction ability to do Iceberg. I think there's a lot of excitement around Iceberg and a lot of people want to move to Iceberg, but I think people are also moving relatively slowly. And I think partly that's because they're running, they're butting their head against some of these problems. So that's sort of my general philosophy background.
On the other hand, there is this huge groundswell of excitement and interest, about Iceberg and for us, it seems like it's pretty ideal because if your data is in Snowflake, and we want to acquire you as a customer, you want to try out MotherDuck. We have to convince you to migrate your data from Snowflake to MotherDuck. If your data is an Iceberg and you want to use MotherDuck, well, we have the same access to that data as Snowflake does. And if we can do things a lot less expensively and we can do things with a lot lower latency, that's actually quite a good thing for us. And, I think there's also some things that were hinted about in Hannes talk. I think he showed a logo and you didn't even say the name, so I'm not even going to say the name. But it is coming out.
Michael Driscoll (29:25)
Can you describe the shape of the logo for us?
Jordan Tigani (29:28)
There's a duck and there's a lake. And, you can kind of imagine them coupled somehow, but that is coming. And I think there are some really interesting architectural things that can come out of that. I also want to take umbrage to the Snowflake driving, the separation of storage and compute because BigQuery did that like three years earlier. And we just sort of didn't pound our chests nearly as much.
Michael Driscoll (30:00)
Right. Well there's a lot of things that Google could market better. True. Kishore, same question for you. You know, and maybe drill in on what Jordan said. And I'll say, in my experience, we all know that, those of us who saw Hamilton's talk today know that one of the beautiful things about DuckDB is that as a developer, you're running so close to the metal, right? You have your data local and those iteration loops are so joyful. Local development remains something that exists. And yet for scale, of course, we have to go to the cloud and for even more scale, we have to embrace things like object storage. If you talk about efficiency and performance as the highlights of Apache Pinot, how are you thinking about cloud object storage and the separation of compute and storage and your cloud architecture?
Kishore Gopalakrishna (30:55)
Yeah, for us, we have been thinking about this for quite some time. I think we built the architecture so that the forward, the storage and the compute can actually be decoupled. But we have been, I mean, similar to Jordan, I really don't know how to solve the use cases that we are solving today with Iceberg. I understand it's so popular and a lot of people are using it, but there is no way you can actually get the latency that you can get with the local storage. I mean, it's done for scanning purposes. It's really good. You add the additional overhead. But when you are looking at millisecond latency, a call to a remote cluster is like 100 to 200 milliseconds. So there is no way you're getting that kind of latency with remote. So instead, what we are thinking is that we actually have the ability to separate the storage and computer at StarTree, but we try to keep our business format instead of the Iceberg format. So we are adding the support for Iceberg, but that's more for when you are okay to trade off some of the things. And where you don't really need that latency. You don't need that concurrency. You don't even need that freshness. I think that's the case where you can actually go for that. But really, the format that we have built is completely optimized for random access and not sequential access. And those are all the things as you get deeper and deeper is just day and night difference. And it's very hard for us to provide that kind of latency guarantee to the users.
Michael Driscoll (32:26)
Sadly, the best storage format doesn't always win in the history of technology, but can totally understand the limitations of Iceberg. Yury, you talked broadly about compute storage separation, but with Iceberg specifically, how is ClickHouse thinking about support for this technology?
Yury Izrailevsky (32:44)
Well, first of all, disclaimer I am partial to Iceberg as well. Toby and I, we worked at Netflix together, and I was involved also with the origins of that. So, I think it's great technology. When it comes to ClickHouse and data lakes. So to your question, why data lakes versus vertically integrated data warehouses? A few years back, the answer would have been, data warehouses are faster, but data lakes are cheaper because it's cheaper to just throw it in an S3 or some object storage. And you're more flexible in terms of ingesting all sorts of data, structured data, semi-structured data, whatever you have. And there's also more versatility in terms of which query engines you can run on top of it. So if you want best performance, you use data warehouses if you want, lower costs or more flexibility and versatility, you use data lakes.
Well, let's see where we are today. And again, things have been changing. You know, Snowflake has integrated Iceberg as a first class and Databricks has more data warehousing capability. But when you look at ClickHouse today, well obviously this is faster to run queries in ClickHouse native storage. And again like decade and a half of investment and the merge tree architecture {and that what really} and skip indexes and everything else that makes ClickHouse really fast. It is impossible to reproduce it today on top of Iceberg. So you do have faster, much faster queries that way. However, because ClickHouse uses object storage as well. And actually the compression that native ClickHouse compression is actually better than parquet, which is a columnar format that's often used in data lakes by about 20 to 40%. It's also cheaper and we don't markup our store. You pretty much pay 20 bucks per terabyte per month. And so it's faster, it's cheaper. You also have the flexibility with semi-structured data with JSONs. Now they are first class citizens with ClickHouse. And we put in a lot of work. And actually, if you want to read something really cool about just an architectural trick, the variant data type of transposing JSON objects to tuples into the column the storage, which is very non-trivial, and how it was implemented. It's amazing. Once we implement that gives us like three orders of magnitude performance improvement. There's actually a benchmark called JSONBench. You can Google it. You can compare the performance on JSONBench of various systems and ClickHouse thankfully is far ahead. So it's cheaper. It's faster. You have the flexibility with semi-structured data. But if you have, if you're an enterprise with a long history of using, I don't know, Spark, Trino, Dremio or whatever other query engines, and you don't want to migrate everything. You can run ClickHouse side by side on top of Iceberg. We support all of the cool features, schema evolution, time travel. Right now we support Iceberg v2. We're working on v3. Eventually you'll be able to write into it. So yeah, it is a first class citizen. And we're definitely betting very heavily on Iceberg. Is it the same performance? No. At least not yet. But yeah whatever works best for you.
Thoughts on AI
Michael Driscoll (36:44)
Okay. Last set of questions and then we're going to turn over some Q&A. I am looking to be thoughtful. I promised the folks who said that their children were the most important thing to them, that we would get them home to see their children tonight before bed. The question, the topic, of course, we would be remiss not to mention is AI and I'm going to frame the question in two parts, which is for each of you, what are the actual real world use cases for AI in your current companies? Where do you see real palpable value? And then secondly, what's your prediction for how this fourth technology revolution we’re living might impact or change the course of your business. So first the real and then the fantasy prediction.
Jordan Tigani (37:50)
So obviously AI is changing. Changing things very quickly. It's changing things super quickly for developers. Everybody's using Cursor, everybody's using MCP servers. And it seems like every week, there's something different. AI is changing or it seems to be changing the data world a lot more slowly than it is the world of software. And, if anybody got a chance to see Hamilton's talk today about Instant SQL, he talks a lot about, hey, there's no real SQL, like, there's no debugger for SQL and the kinds of tools that data analysts and data scientists have been using are trapped in the 70s for a lot of use cases. That said, there are certainly ways that we can apply AI to make the process of data analytics better. A lot of people talk about how Text-to-SQL is fundamentally broken without a metrics layer. And that's why a lot of people are excited about metrics layers.
Michael Driscoll (38:59)
It's really great point you made there. Metrics layers are great.
Jordan Tigani (39:04)
One of the things that we want to do, actually, we've seen people using Text-to-SQL in constrained schema situations like people building embedded applications like analytical applications, they have a single schema for all their customers. And, they can have very, very clean semantics on that schema. And you can get actually really good Text-to-SQL performance. But if you want to solve the sort of the general nasty messy data warehouse problem where you have, I think you were talking earlier about latest “table underscore V3 final final”, and that has the same schema as you know 17 other tables. It's very, very hard for AI to know. You know what you know which table to pick, let alone how you compute whether your fiscal year starts in February. I do think that there's going to be a lot of opportunities for tools to help with this, a lot of opportunities to sort of just help automate certain things and make the process of writing queries a lot more delightful, like automatically filling in defaults automatically, really, really good autocomplete. There's also some things with better context windows and probably the ability to actually generate better SQL, and better metrics layers. But I think there's also a lot of opportunity in that space. I think anytime things aren't clear, it's an opportunity for somebody to come in and do things better. I've certainly talked to a bunch of founders that are working on interesting things in the space, but I don't think this is certainly an area where I don't think anybody has conclusively solved the data problems with AI.
Michael Driscoll (40:58)
I'm going to prompt Kishore because we've talked about this in our Data Talks on the Rocks interview.
Kishore Gopalakrishna (41:03)
Since most of our users are using user facing applications, that means they are building applications for their end users and their people actually do click this, then go do this, then click this. I think a lot of these tools are actually becoming conversational apps. So it's actually lowering the bar of who can actually access this. Because even if they have a very nice dashboard, you still have to learn something like, hey, if I need to answer this question, I need to go in so many different places. So it's actually pretty interesting. Some of our customers have just thrown away their dashboards, just built a conversational app on top of it. And I think to Jordan's point, it's actually possible in our case because it's restricted. It's very well defined in terms of the schema and the metadata that you provide. You don't have “final final final” table. So it makes it a little bit more kind of constrained in terms of the problem. So it's one thing that's actually very interesting.
Michael Driscoll (41:59)
I think it's a point for all three of the engines that you are the database technologies you've built, there's the speed. And when we talk about conversational AI agents, a core tenet of intelligence is that if I ask you a question, we don't have to wait 60 seconds for an answer. So all the potential for using agents requires having a fast backend, to be able to serve that. Yury, when you and I were talking before, you'll get the final word on AI, and then we'll open up for questions. You showed me this thing that I was amazed by llm.clickhouse.com, which is an AI agent that lets you interrogate some public data sets and ClickHouse. Tell me broadly about how you're thinking about AI.
Yury Izrailevsky (42:45)
When you think about AI, it's a very overloaded term, right? I mean, sometimes you think about big AI companies, and most of them, if not all, are using ClickHouse. You know, Anthropic, OpenAI, LangChain, DeepSeek. We talked about Weights and Biases, so forth.
Michael Driscoll (43:05)
An insecure ClickHouse, just to reference DeepSeek.
Yury Izrailevsky (43:10)
Don't use DeepSeek. Only use those who are using ClickHouse Cloud. DeepSeek is not one of them. And then a few years ago, I meant training models and running inferences. And there's still a lot of customers who are using ClickHouse. We have RunReveal doing security analytics and they actually built an MCP server on top of their files. And Ramp, an expense management system, they're doing vector search on ClickHouse and it's built right into the database. So you don't have to copy data out into a separate vector database. You can do all of that there.
But just looking at the audience here, how many of you guys have trained or ran an inference on the model in the last 30 days, let's say, raise your hand. Okay. So there's maybe 3 or 4 people. And then how many of you have used an AI agent? ChatGPT or anything else. Everybody. And even if you didn't raise your hand, I guarantee you used a customer service app or anything else, I guarantee you used an agent.
So I mentioned llm.clickhouse.com. That's a great example of what you can do with the semantic interface through an agent. If you have your phones or your laptops, you can open them up. Just go to llm.clickhouse.com. You don't need to have an account with us. You can go right now. And what we did is we preloaded a bunch of open source, publicly available databases like the GitHub repository history or the New York taxicab rides or UK property data set. You can go to the prompt and say, show me the top ten most expensive cities in the UK using the colors of the British flag. Type it in. Go ahead. And you'll see it. And it's actually connected to Anthropic Claude 3.5 Sonnet. And it shows you all of the query. So it translates. It understands. So the only context we're passing on to them is the schema. And it's smart enough to figure out which table you're referring to, and how to set up the query. It tries as if it doesn't work, it just tries something else. It's amazing. Or go to GitHub and ask, how many pull requests for, I don't know, DuckDB, Apache Pinot, and ClickHouse and it tells you all of that stuff and, within ClickHouse, we've completely moved to agents. I have not looked at a BI dashboard in probably two months because we have an agent that again connects to it and it's like once you try it, llm.clickhouse.com, it’s amazing. And we do it for a knowledge base. We do it for other things. And, I think that's really in front of us. The future at least, in the near-term is building those agents, integrating them, putting them in front of databases, connecting them to various applications. I think that it's going to be driving a lot of the usage.
Michael Driscoll (46:21)
But I think it's important that these are the kinds of things that only work with fast, real time databases. It's much harder if you're running these queries on Athena.
Yury Izrailevsky (46:30)
If it takes 15 minutes to come back, you will not be a very happy user.
Audience Questions
Michael Driscoll (46:36)
They lose your patience. Okay, we have a few minutes. I'm going to open it up to, if anyone has been holding a question that they'd like to ask of Yuri, Kishore and Jordan. First I want to give a round of applause for you all.
Audience (47:02)
So are you worried about having an open source software that then Amazon or Google or Microsoft just steals and distributes it to the kajillion people who are their customers as opposed to using your kind of cloud service?
Michael Driscoll (47:18)
Okay, we're going to have to direct that and I'll do the directions. So the question was, are you worried that Amazon, Microsoft or Azure will just steal software? Certainly we saw that was a greater fear, I think a few years ago when Elastic and Confluent changed their licenses. But I'm going to ask Jordan because I think I have an answer already for ClickHouse. Jordan, how are you going to prevent Amazon from just starting? I don't know, Amazon Duck.
Jordan Tigani (47:42)
So a couple things. One is the way we integrate with DuckDB is we're integrated inside DuckDB. So you change your database name to start with MD colon and it reloads our extension and starts talking to MotherDuck in the background. And so, if Amazon is running a DuckDB server like they won't have the ability to do that. There's a bunch of mechanisms that we've built around DuckDB. DuckDB is an embedded database, it's not a data warehouse. All the sort of user management and sharing and scaling and all these things that Amazon or Google would have to build around it. So it's all code, somebody could do it. But it's not like you just sort of take the service and run it in a Kubernetes cluster or container and then you're done. So we're not particularly worried about that, partially because of the architecture. Partially because DuckDB doesn't provide a lot of these features. And then finally, the more people who use DuckDB, the better it is for us. And like we would love to see the DuckDB community grow larger. You know, Elastic, they certainly hurt their business. The fact that Amazon built a competing service. But, you know, they're doing okay.
Michael Driscoll (49:07)
I'm going to move to another question. But I think in general, what we've observed is that the hyperscalers are now more friendly to some of these, they realize they make money, whether people use Amazon Elastic or they use Elastic Cloud, it's still compute that they can charge for. Other questions.
Audience (49:27)
Michael, given that, what you just described with llm.clickhouse.com, I'm very curious about what the future of interfaces, BI interfaces is? I'd love to hear your perspective.
Michael Driscoll (49:47)
Well, it's a great question. I'm happy to answer it. I think the future of BI interfaces is I do think a lot of this is predicated on having real time analytical databases speed behind it, I believe certainly. Well, agents are a great way. Natural language is a great way to prompt folks. I do still think there's a lot to be said for tactile interfaces. I often find that pointing and clicking can actually get you an insight that sort of describing in natural language what you're looking for is not as effective as dragging and zooming and drilling and pivoting. So I think we're not going to say goodbye to all of the visual interfaces that have been created. And if that were the case, I think that company narrative that just had, oh, we'll just have a little pen that we talked to, it turns out people still like their phones. They still like to point at things and swipe. So I think the tactile interfaces have a place. So will the sort of natural language interfaces for analytics. I think both of these can kind of coexist. But thank you for that question. Other questions from the audience.
Audience (50:56)
I'm curious, how do you see developments in terms of the database schema given LLM’s specific ways of handling data. For example, I tried llm.clickhouse.com, so the queries, the list of databases, the list of tables, etc. takes quite a long time. So probably would be more efficient if it was preloaded with information about what data the specific ClickHouse database contains. Instead of just querying all the schemas that can be directed towards querying. So do you see any specific, tighter integration between LLM and databases in the future? Because that would allow for newer use cases.
Michael Driscoll (51:43)
Great. And if you can summarize the question Yury before you answer.
Yury Izrailevsky (51:46)
So the question is, if you go to llm.clickhouse.com because we're only passing the schema, is the context, it actually has to figure out which schema to use. Obviously you can pass a lot more stuff in the context. And if you use the copilot in the ClickHouse console for the SQL editor, we're actually passing more information. Again, we’re being very careful not to share customer information, but there's more information about the ClickHouse service that it goes to in that case GPT4 and there you see much faster, but it is really the judgment call of the developer of this agent of how to set it up and how much context to pass.
In this case, we were actually quite surprised. We were expecting that in addition to the schema we would need to provide more stuff. But, Claude just figured out how to interpret and how to adapt very, very well. It doesn't seem like it needs a lot of additional help. Yeah, it takes a little more time, but I actually like that. It shows all the homework, it shows all the queries, and it's actually kind of a good way if you're not a deep SQL expert. And I don't consider myself a deep SQL expert. I'm more of a distributed systems person. I think it's great. I'm actually using that to learn how it thinks and how to optimize things. But yeah, there are definitely ways. I hope we provide an additional context. You can speed things up and provide additional shortcuts.
Michael Driscoll (53:24)
Okay. I'm gonna take one more question. I see a hand raised, over there. Okay. Final question. You, sir. Go ahead.
Audience (53:32)
This one is mostly for Jordan about how most people's data sets are very, very small. Relatively small. I'm curious, one of the things that I've observed, when talking to customers, is that they kind of don't want to admit that to themselves. They don't want to be the small data guy. How are you guys running into that? And how do you handle it?
Jordan Tigani (53:58)
So the question was how do you handle people who think that their data is bigger than it is or people who think that their data is going to be bigger than it actually is? So, I mean, one of the ways is just around education, for example, like the amount of hardware on a commodity, you know, EC2 instance, that's available in every, every region is not super expensive. 192 cores and a terabyte and a half of RAM. That's roughly the equivalent of the amount of hardware in a Snowflake 3XL. Snowflake 3XL is $1 million a year. So if you're using less than a Snowflake 3X, your workload will fit in one machine. So it's like, yes, okay, maybe you do have some big, big workloads.
The other thing is our tenancy model. We can run a, we want a single instance per end user. So you might have a thousand users in your company. You each get a Snow, the equivalent of a Snowflake 3XL. You know again that's a lot of hardware that we can throw at various problems. There is certainly a kind of mental block. And that's one of the reasons why we kind of poke light at, you know, where the boundary is between smart small data and big data. But I think that boundary is receding. Every year these machines get bigger and bigger is sort of the thing that used to be really complicated distributed systems. Well, you can have twice as much data, and then the next year is twice as much data, and then pretty soon, you may not need those complicated distributed systems.
Michael Driscoll (55:38)
Okay. I think we're going to call it, Marianne, tell me how long do we have for people to relax and hang out if they want to? Okay. So we've got about 45 minutes if people want to come and talk to these amazing founders, we'll give them a round of applause. Thanks for joining us and enjoy the rest of the night.
Post-modern ETL chat with the founder of Tobiko Data
Michael Driscoll
So first, what do we have in store tonight? We've got a double feature. First, we're going to be talking about what I'd like to call the post-modern data stack vendors, Tobiko Data being one of them. And then after, we'll take a quick break, people can get some fresh air, and then we've got an incredible group of next generation post-modern database founders, real time databases - MotherDuck, ClickHouse, and StarTree. All three founders will be joining us on stage. Super excited for that.
Why am I here and what brought me here to host this event? Well, Rill, we're BI tool. Some of you may be aware of what we offer. We are admirers of what Tobiko Data is doing with SQLMesh and SQLGlot. We're inspired by their work. We're also heavy users of DuckDB, Apache Pinot, and ClickHouse. Of course we've got StarTree and MotherDuck representing those two open source projects, DuckDB and Pinot, here with us. We love these tools and we think they're changing the data landscape. We're not going to tell you any more, but you can check out rilldata.com if you want to download an amazing BI tool. Of course, you've also got great tools out there like Hashboard. Carlos, we won't forget about you either. Check out hashboard.com as well.
Okay, my guest tonight to start things off is Toby Mao. Toby, I'm going to just get right into it. You were an engineer at Airbnb. You were one of the engineers working on a very popular project at Airbnb called Minerva, which is a metrics store or a metrics layer, that was successfully implemented at Airbnb. Now, fast forward a few years, you're the founder of Tobiko Data. First tell me how did you go from a metrics layer, you know, leading this very successful open source tool at Airbnb to a dbt, arguably a dbt alternative in, SQLMesh and of course, supplemented by SQLGlot. So I just want to hear a little bit about how you got here.
Origins of SQLGlot
Toby Mao (02:11)
Sure. Yeah. So I started at Airbnb leading the analytics team that included both metrics, Minerva, and experimentation. And, when I started, Minerva was quite prolific, but it had a lot of problems, like it was very YAML based and there was no SQL understanding, it was very slow. So part of my work there was to help design Minerva 2.0, which took things like single parsing and a lot of improvements to the metric definition layer. So as I was working there, I was thinking about starting a company, and originally, the co-founders of Tobiko Data, we were discussing and we were thinking about actually creating another metrics layer. But at that time, around 2022, there were some other kind of metric layer companies and they weren't seeing much traction. So we kind of went back to the drawing board. And I realized that…
Michael Driscoll (03:13)
By the way, one of those other companies came out of Airbnb, didn't it?
Toby Mao (03:17)
Sort of. So the co-founders did work at Airbnb, but then none of them actually worked on Minerva. Okay. They just used it. But I realized that even though Minerva is known as a metrics layer, the true power and what made Minerva successful was that it was a democratic transformation framework. Because without good data, you can't have good metrics, right? Bad data in will be bad data out. And so Minerva really empowered analysts and scientists to be able to very easily define large scale transformations, to then consume in the metrics layer.
Michael Driscoll (03:59)
And SQLGlot, was there something like SQLGlot at Airbnb that was sort of, from my experience when I was at Snap was that they also created a centralized metrics, governance group, and they had actually built, I think this is a bad architectural decision, that they built something in Java to express all of their metrics, definitions in Java. Obviously SQL is a much better language to express metrics. At Airbnb, what was the sort of core language for expressing metrics at Airbnb, like a metric like MAU or…
Toby Mao (04:39)
So before I joined, it was all in YAML, Jinja and YAML, and some kind of boolean algebra language. It wasn't great. And so part of my inspiration or what I wanted to do was I wanted to leverage SQLGlot, which I had built during my time at Netflix. I brought that in.
Michael Driscoll (04:58)
Okay, so just so we know, SQLGlot preceded Airbnb. You built it at Netflix and was it open source?
Toby Mao (05:05)
Yep. So at the end of my tenure at Netflix, I started work on a side project because I knew companies like Netflix, they use many different engines, they use Spark, they use Presto, Trino, Druid, and scientists will write queries and want to run it across both, right? They want to run it in Spark if they're trying to do a reliable ETL process. They'll want to run it in Trino or Presto if they want something fast. But they're not compatible. And so I originally created SQLGlot in order to be able to run queries across both Spark and Presto.
Michael Driscoll (05:39)
So did Minerva 2.0 leverage SQLGlot?
Toby Mao (05:42)
Exactly. That was one of the main innovations, is that now we could have a better language we could run. Also, the queries were all written in Hive, and we leveraged SQLGlot to run them in both Spark and Trino. So they got that ability so using SQLGlot was one of the big architectural decisions of Minerva 2.0.
Origins of SQLMesh
Michael Driscoll (06:00)
So let's talk a little bit about SQLMesh. So SQLGlot, you created this as a side project that came out of some work at Netflix. You took it with you to Airbnb to build Minerva 2.0 their metrics layer. Tell us about SQLMesh and the origin of that. That's kind of the other major technology at Tobiko Data.
Toby Mao (06:20)
So we ended up choosing to build a company around transformations. And kind of the inspiration for that was so Minerva was a big transformation tool at Airbnb. And while there, I heard about dbt and I got a demo of it from some excited analytics engineers. And when I saw it, I had a lot of questions. So the first thing I asked was, well, what if I just want to run one day of data and they're like, you can't do that. You have to just refresh everything. I was like, really? But you can't just refresh everything. And maybe that's because there's too much data. And so that kind of question led me down a path of researching what dbt was, and realizing that there was a big opportunity here to basically blend understanding of SQL having state and all the advancements of understanding how to do two data deployments. And ultimately that's what created SQLMesh.
Michael Driscoll (07:13)
So maybe there's double click on this, this may be the biggest question that people would ask when they're, you know, a lot of folks in the audience here are developers working, building pipelines, making choices about databases. We'll talk about databases of the next group. But, a lot of folks are going to say, hey, I've heard of this thing called SQLMesh. I also know this thing called dbt. Why should I choose SQLMesh? You know, this young startup in the Bay area with these interesting ideas? Why should I choose SQLMesh over dbt? I mean, nobody ever got fired for dbt running on Snowflake.
Fundamental design and architecture choices
Toby Mao (07:50)
Fundamentally they're designed very differently. dbt is great, don't get me wrong. But it's really stateless, there's no state and everything is just a script. And you just kind of run your Jinja SQL. So if you're familiar with the DevOps space, it's kind of like Chef, where you can tell it exactly what to do and it just does it blindly. SQLMesh on the other hand, is stateful. It understands SQL and kind of is more declarative. So it's more like Terraform. And so there are two very different approaches for transformation.
Michael Driscoll (08:20)
Let's talk about the incremental modeling. I think that if any of us who work at scale with data knows that dropping and replacing multi-terabyte tables is a no go. Clearly Airbnb did not have the luxury of dropping and replacing multi-terabyte tables. How does SQLMesh deal with incremental data in a way that's different than others? And having read your documentation, it's clear that you've put a lot of thought into these incremental pipelines in particular.
Toby Mao (08:53)
So the tricky part of incremental processing, you know, one day or a month at a time, is that you have to understand what has been done and what needs to be done. Things like Airflow and other tools have been doing this for a long time. But since dbt has no state, you can't really do that. And so in dbt, in order to do incremental processing, it's really left up to the user. You have to do a select self query to kind of figure out your last time stamp and then get all the data after that. That's why you can't just get one day of data. You have to get all the data past a certain time. But since SQLMesh actually stores date and understands okay, you process all of January, you need to process February. The user just says, well, I want all the data between start and end and then SQLMesh will populate start to end. And this is very similar to tools like Airflow or Dagster where you have a first class understanding of time.
Michael Driscoll (09:41)
So, I downloaded SQLMesh and started playing around with it. One thing I noticed, tell me if this is right, it seems like you have this the first version that developers work with. DuckDB is a pretty integral part of SQLMesh, and yet the pipelines that you write in SQLMesh can be run in ClickHouse. They can be run in Snowflake, they can be run elsewhere. Just tell us a little bit about what role this embedded database plays in SQLMesh, and how you're able to then deploy these pipelines to different engines out there.
Toby Mao (10:18)
Well, there are two parts of it. One is we wanted the initial Hello World to be very easy to set up. No configuration. So DuckDB was an obvious choice because once you pip install it, you have a full fledged analytics database, and you can run anything. So that's one of the reasons why DuckDB is like the kind of starter.
Michael Driscoll (10:37)
There is also a tool called chDB out there which is also an embedded ClickHouse.
Toby Mao (10:43)
That wasn't out when we created SQLMesh first. The second part of DuckDB is that we actually leverage it for unit testing. So a lot of companies use SQLMesh in BigQuery, Snowflake and Databricks as their main pipelines. But when you want to run unit tests, so I'm not talking about data quality checks like nulls and uniqueness, I'm talking about actual data unit tests. We're given a fixed set of rows, you're expecting a fixed set of output rows. Because of SQLGlot, we can take any BigQuery code, Databricks code, and convert that into data in DuckDB. And so that would mean that without an internet connection you can do all your CI/CD very quickly and effectively.
Michael Driscoll (11:25)
What are the database dialects that are supported today and what's on the roadmap? If we were to sort of say for folks out here that were running different databases and thinking about this ability to write almost database agnostic pipelines, what does SQLMesh or SQLGlot support today and what’s in store in the future?
Toby Mao (11:44)
Well SQLGlot supports over 20 dialects, I can't name them all, but SQLMesh supports all the main ones. You know, Snowflake, BigQuery, MySQL, Postgres, T-SQL.
Michael Driscoll (11:54)
What's been the most painful SQL dialect that you've had to support? In SQLMesh?
Toby Mao (12:00)
I'll plead the fifth.
Michael Driscoll (12:02)
I plead the fifth. We love them all. They're all beautiful databases, as long as they sound...
Toby Mao (12:07)
I will say, though, that someone got very upset at me for not supporting Greenplum. So we do not support Greenplum.
Michael Driscoll (12:14)
Well, I think I saw that in your community Slack channel that someone was asking for Greenplum. It's very old. Isn't it just Postgres? Greenplum is a fork of Postgres.
Toby Mao (12:26)
A lot of them are.
Michael Driscoll (12:28)
Okay. Well, speaking of different tools in the ecosystem, I think another question is, what are the complementary tools, if a customer comes to Tobiko Data and says, we like your ideas, we love this idea of an engine agnostic pipeline framework. There's Astronomer, there's, of course, the commercialization of Airflow. You've got Dagster, you've got Kestra. How do you play in this? I'm going to call it the postmodern data stack, because we're trying to reduce the number of vendors we all have to pay SaaS fees to. What are some of the other complementary products out there and maybe kind of adhere to label categories? We've got orchestrators, we've got data contract folks. Who do you feel like you complement pretty well?
Toby Mao (13:17)
It's very common for larger companies to have multiple tools. And so they might have Airflow and dbt and SQLMesh or something like that. So SQLMesh works very well with those main orchestrators. We have first class Airflow support, Dagster support. So with Tobiko Cloud, which is the cloud enterprise version of SQLMesh, you can easily connect those two, listen to signals, show all your DAGs and all those kinds of things. We also do have integration with Kestra. I believe they've integrated that into their whole stack.
Michael Driscoll (13:54)
So I'm gonna ask this question to our real time analytics database panel in a few minutes, but I think the, the question that a lot of folks have is, and we've seen this, I'll just say we've observed it with dbt, amazing adoption among the world of data practitioners. But over time, there's always this fear that the open source product, you know, gets fewer and fewer things are being put into the open source product. And eventually, they start raising prices on some of the offerings in the cloud. This is a two part question. First, what is the difference between the Tobiko Cloud versus we want to call it the core, open source projects of SQLMesh and SQLGlot? And then secondly, how do you intend to anticipate and avoid the disgruntled users, when in the future you make some choices about the commercialization of your product in the cloud?
Toby Mao (14:55)
Yeah, that's a good question. And I think for us, I mean, if you look at our history, you look at our actions, I mean, it's pretty clear that we're very committed to open source. I mean, SQLGlot has been a passion project of mine, and now the company runs its license. It's MIT. SQLMesh is Apache, but if you look at the kind of features that we have for SQLMesh, we have state, right? Something that other companies have said would never be part of open source. I mean, that's a big thing, right? We have scheduling, right? We have a UI, right? All these kinds of things, SQL parsing, right? All these huge features that a lot of companies call-level lineage right in open source already today. We are really giving so much more than others. And so I think, you don't have to hear my words. You can just see what's out there. Now in terms of how cloud is different.
Our philosophy is that cloud should enhance a great open source product. But we want open source to be powerful and usable by anyone and we want cloud to extend that. And so for companies that are looking for more to have, like enterprise grade operational reliability, you want cloud. And so our cloud offering has things like managed state managed infrastructure, managed scheduling, integrations with other schedulers, observability, better Dag management, role based access control, security and all those kinds of things.
Michael Driscoll (16:24)
The last question is on the shape of the offering. There's sort of today, two common models of delivering cloud services. One is managed SaaS, the Snowflake model, how I refer to it. Then there's the Databricks model, which is bring your own compute, which one is for you today and why?
Toby Mao (16:41)
We actually do both. So the starter tier is all cloud. So we manage everything, but we work with data. And so data is sometimes very private. And so we offer hybrid as well, which means that you can run containers that connect to our cloud. So our cloud is just metadata. And then all the actual data processing Python and SQL have it on your own VPCs.
Michael Driscoll (17:05)
Toby, thank you for being here. Thanks for telling us about this incredible open source product that you've created. And, and we wish you the best for a very successful future.
Toby Mao (17:14)
Thanks for having me.
Ready for faster dashboards?
Try for free today.